People with a doctorate may recognize this moment: you realize there are few souls in your direct vicinity who have their feet so deep in the exact same ‘mud’ as you. You effectively ‘marry’ your topic for four years, for better or worse, bouncing your ideas off the wall, and the occasional colleague who researches ‘something’ in a similar direction. Hence, while I was working on my own PhD some time ago, I jumped at the opportunity to attend a conference in my subject area. Hoping this science Valhalla would be swarming with open, kind and like-minded folks. Once I was there, I learned this was only partially true.
A Lunch Conversation
On the first day, during lunch, I talked with the person seated next to me. The conversation roughly went like this: “I published three times already at conference A, twice at conference B and I have an outstanding publication for journal C. I am not even in my third year yet and I already have nearly six publications.”
I frowned as I struggled to respond and carry the conversation forward.
Science is filled with humans and boasting is a very human behavior. It is not the main thing that I found off-putting. What really bothered me was that, as we continued to chat, it became apparent that this person seemed completely detached from his research. He was not intrinsically curious or motivated by what he was working on at all. His main drive appeared to be the reward of getting published.
This disappointing conversation got me thinking about incentives, creativity and drive. While my experience is limited to computer science, I think this topic is as relevant today as it was back then. How do rewards incentivize behavior in science? Have we set things up to yield what we want, what we need, what we expect?
The Dripping Candle
In 1938 Karl Duncker ran an experiment intended to measure creativity. He gave every participant a candle, matches and a box with some pushpins. He then asked them to fix the candle to a cork wall upright and light it. Additionally, he demanded this happen in a way where the candle’s wax would not drip onto the table below.
The task generally took his participants a little while, but most of them came up with the right solution within five to ten minutes. Which is: empty the box with the pushpins, fix the box to the cork wall with some of the pins, put the candle in it, an finally light it. To come up with this solution, participants had to literally ‘think outside’ the box. They needed some creative insight to see the box can be used for a different purpose than holding on to those pushpins.
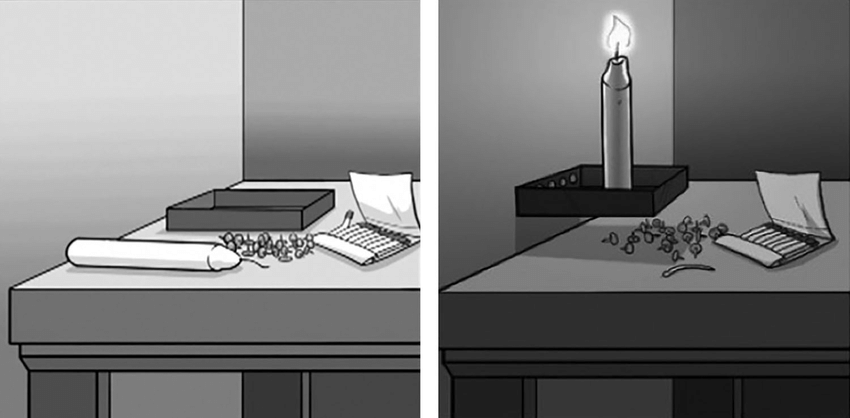
The Other Condition
Duncker created an interesting condition in this experiment where he would offer money for coming up with a solution faster. The fastest participant would get the most money. You might expect people to come up with a solution faster when clearly incentivized to do so. However, that is not at all what Duncker found. Participants that were offered a reward took, on average, three and a half minutes longer to come up with the solution!
Duncker’s experiment shows something interesting about human nature. Namely, that the outlook of a reward can trap us in tunnel vision. It becomes harder to think outside the box, since we actively look for the least costly solution that gets us the promised reward. This blinds us from seeing and trying other viable avenues: it makes us less creative.
A study comparing creative works by commissioned artists with non-commissioned works further confirmed this phenomenon. The commissioned works were rated as being significantly less creative [1].
You may be wondering at this point how this ties into science as a process. For that we first need to understand how science works and how publishing ties into it.
Science as a Process
A scientific inquiry ideally starts with a question rooted in fascination. For example: finding out why something happens in a particular way, how to overcome a specific challenge, or how to further improve an existing approach. Experimentation is all about trying out ideas to reach that goal. Science is messy. The road of ideas traveled is often curvy, may involve backtracking and changing the goal itself. Even if a later rewrite of the narrative commonly suggests a straight(forward) path to a particular discovery.
The ideas to explore usually build upon work previously done by others. What sets science apart is that these ideas can be formulated as statements that can be proved or disproved. The outcomes of a scientific experiment are not mere opinion, but based on empirically supported facts. Repeating an experiment to confirm the outcome further bolsters objectivity.
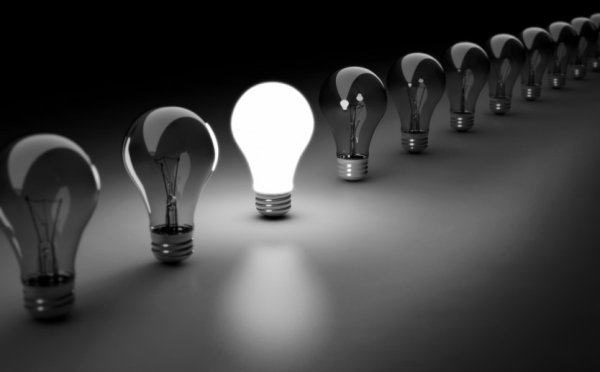
To make this concrete: let’s say that your statement is that all roses are red. If you actually go out into the real world and find some roses, you’d quickly find out that this is not true: there are non-red roses. Hence, you can disprove the statement, referred to in scientific terms as falsifying an hypothesis. Besides pure observation, there are many other ways to prove or disprove such statements depending on subject matter and available methodology.
Back to the scientific process: once a scientist has proved or disproved a statement, she can write down the idea, experimental process and conclusion in a scientific paper. She can then publish this paper, so other scientists can read it, attempt to reproduce it, and build on top of it by citing the paper. This completes the cycle. It is the publishing process where things really get interesting in terms of incentives.
Publishing in Science
Before a scientific paper gets published it is commonly peer reviewed. This means that other scientists, knowledgeable on the paper’s topic, evaluate the quality, scientific rigor, and novelty of the paper. The peer review process can be quite involved. Scientific papers usually require multiple review rounds, if they make it to publication at all. It is not uncommon for top-tier conferences or journals to reject eight out of every ten papers submitted. All of this is intended to make sure that only the highest quality papers actually get published. However, there are several problems with this approach to science.
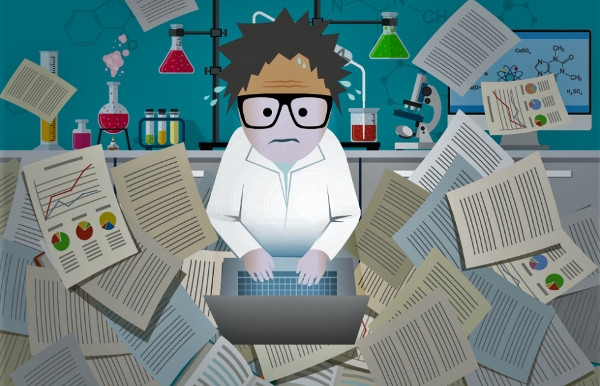
Firstly, there may be more good submissions than there is space at a top conference. The rejected papers go through a procession along ‘lower-tiered’ conferences or journals, until someone accepts them or they end up unpublished.
Secondly, the reputation of a paper’s authors influences the chance of acceptance. This can be mitigated with double-blind reviewing: the authors don’t know who their reviewers are (as is common), but the reviewers also don’t know who the authors are. This system is not ‘airtight’, as the research community around specific topics is usually small.
Thirdly, reviewers differ in quality. Sub par papers may be unduly accepted or decent papers may be rejected for strange reasons. For example: I once had a paper rejected because it ‘reminded’ the reviewer of something he read before. He did not provide a reference to that work. Such low quality reviews are problematic, and are often only apparent after the fact.
Peer review is important, but only one factor in scientific publishing. After publishing, something else starts to play a larger role. Perhaps larger than it should be.
The Power of Links
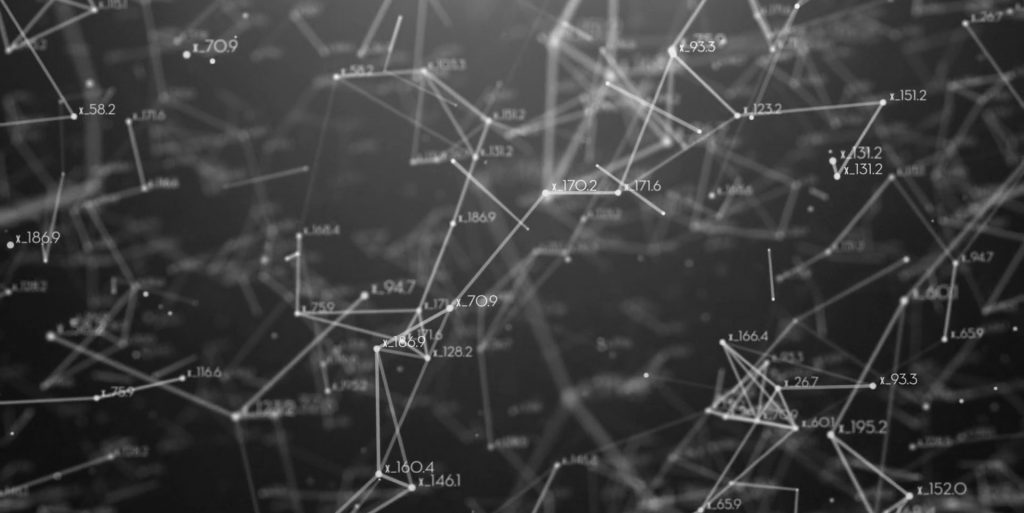
The innovation that ensured Google’s rise to fame in the late nineties was that they extended search with a completely different approach than all of their contemporary competitors. The then common approach was to simply search through webpages by looking for the search terms users entered. Google additionally heavily factored in how many times a link to a particular webpage appeared on other pages. Effectively these incoming links from other pages functioned as ‘votes’. Pages that both matched the user’s search terms and won the ‘vote’ by other pages, formed the top search results. This yielded superior search results over just matching the search terms [11].
Scientific citations, one paper referring to another paper, similarly form links. Hence, the same logic applies. When a paper is cited many times it is likely to be important. If an author has many cited papers she is influential. When a conference’s or magazine’s papers are cited many times it becomes the top in its field. These numbers indeed exist. For individual scientist the h-index is often used. It works as follows: the more a scientist publishes and the more she is cited, the higher her h-index. There are other related indicators, such as the impact factor commonly used for journals. These kinds of indicators attempt to capture productivity, citation reach and importance for purposes of analysis [4].
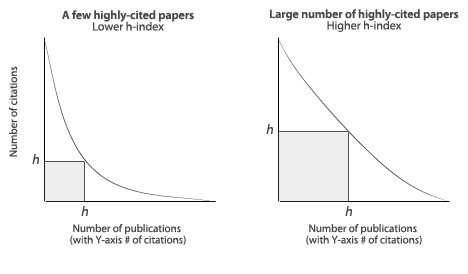
A Dark Science Investment Experiment
Let’s descend a bit into a dark thought experiment.
Say you are a funding agency and have some money to invest. You want to fund a scientific endeavor by investing somewhere where it pays off. Since looking at scientific work qualitatively requires a lot of effort, looking instead quantitatively, at numbers, seems seductive. You are looking for a proxy: who produced the best scientific output? You’d want to invest in a safe bet, which means reputable scientists. Or at the least: you’d want to know what risk you’re taking so you can ‘spread your bets’.
The reputation of scientists can easily be found using those indicators that express influence. The h-index, initially an analysis tool, becomes a selection tool in the hands of funding agencies such as yours’. Recall that this h-index metric started off as an innocent tool to look at the past and uncover who was influential. However, now it inadvertently turned into a goal optimization metric for scientists wanting to attract funding from you.
The Risk Aversion Dance
You could view the h-index as a proxy for a scientists’, conference’s, or journal’s reputation. A higher reputation yields more investments. Perversely, this results in the reputable becoming even more reputable. This snowball effect potentially leads to the emergence of a scientific status quo.
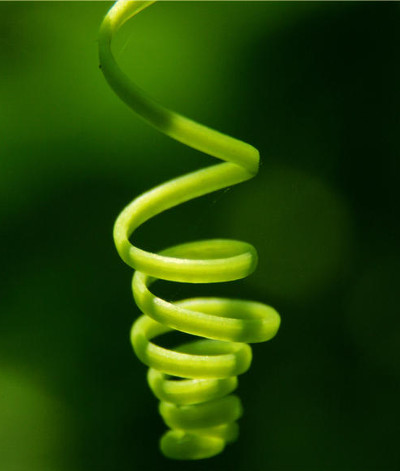
Taking the scientist’s view: they need to convince either funding agencies or companies to provide them with money to carry out their research proposals. A dance that many scientists conduct reluctantly. They’d rather focus on doing actual research than spin a narrative.
Scientists want to ensure their proposals get funding, providing an incentive for safe plans that fit within contemporary scientific thinking. Similarly, the funding agencies looking for a sure bet, will go with safe proposals.
We are left with a system intended to promote innovation, that instead seems to discourage it. This should not surprise us. As Duncker’s experiment showed, the objective is no longer the pursuit of a solution for its own sake, but rather the pursuit of a measurable reward. From the investors perspective: a proxy for the return of investment, and from the scientist’s perspective: a proxy for funding. What kinds of behavior does this set up of incentives induce?
Fraud in Science
In 2011 Dutch university professor Diederik Stapel was at the apex of his scientific career, with over 130 attributed publications. He made headlines with bold research. For example: with an experiment that showed that meat eaters were more selfish than vegetarians. However, in mid 2011 several of his PhD students chimed the alarm.
The students suspected their professor had fabricated data for some experiments. While Stapel scrambled initially to defend himself, he later fully admitted guilt. He had started this practice years before, after writing a string of papers that got little attention. His approach: reversing the math to get to ‘sexier’ outcomes that still looked plausible for research on the periphery of the field.
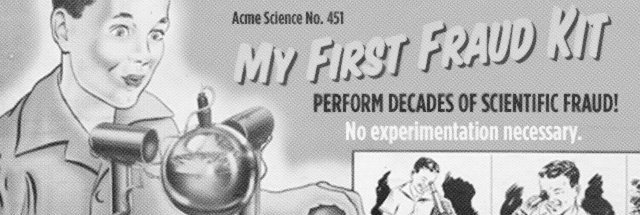
Stapel claimed that his lifelong obsession with elegance drove him to fabricate results that journals found attractive. He reflected on his behavior as being an addiction where he sought to get away with increasingly more extreme fabrications. An investigation that took years revealed that at least 55 of his 130 publications needed to be retracted due to suspicions of tampering. After becoming one of the main faces of large-scale scientific fraud, Stapel wonders when the time comes that he has atoned enough [3, 7, 8].
Stapel’s case is easy to dismiss as outlier. Just one of the few souls who strayed from the righteous path. However, that would be far too easy, as he was not alone in his deviation. Yoshitaka Fuji, Marc Hauser and recently Joachim Boldt, among many others [9]. However, just calling out names is easy and distracts us from examining the deeper root causes. Fabricating data is obviously wrong, harmful and can set back scientific fields for decades. The relevant question is: how can we set up things better to prevent it? And, what about more subtle cases?
“I find the replication crisis in social psychology fascinating … How can such a crisis emerge, if everyone abides by the rules? Do they all commit fraud? This seems unlikely, but how do we then define pristine science? What is moral?”
– Diederik Stapel (2020) [7]
Systemic Biases
Large cases of scientific fraud may remain buried for years. This is a failure not only of the scientist in question, but also of the scientific community. Fortunately, once identified we can at least pick out and invalidate the problematic publications. This is not true for other much more subtle effects that permeate scientific publications. Our only resort is repeating the described experiments to reproduce the results. This is often hard. Dorothy Bishop has identified what she calls the four horsemen of the reproducibility apocalypse: publication bias, low statistical power, p-value hacking and HARKing. Let’s look examine these [2].
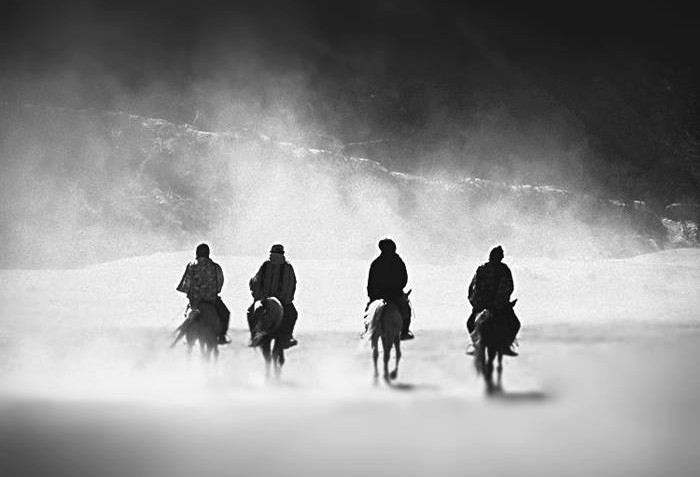
Publication Bias & Low Statistical Power
Firstly, there is the case of scientific experiments which fail. The initial hypothesis (a statement) could not be proved. Authors have a much lower chance of getting papers about such experiments published. In turn researchers are less likely to write them up and conferences and journals less likely to accept them. This publication bias leads to unnecessary repetition of experiments at best, and well-intended overoptimistic interpretation of results, to mitigate failure, at worst [10].
Publication bias is endemic and will remain so as long as sample sizes commonly used in research are too small and the methods used to assess adequacy of sample size are deficient.
– R. G. Newcombe (1987) [12]
Secondly, conducting experiments on too small a scale, or with too little data. A too small sample size can lead to either accepting or rejecting the hypothesis based on insufficient evidence. Though, the bias is towards accepting too small studies with statistically significant results [12].
P-value hacking & HARKing
Thirdly, the p-value, a statistical metric that tells us how surprising an experimental result is if you would not be looking for it. Essentially it expresses how likely it is that a result is due to chance. A low value means that a chance effect is unlikely, and thus that the result of an experiment is statistically significant. A commonly used upper boundary for this value is five percent. This hard upper boundary forms an incentive to repeat the experiment, pick favorable trial outcomes, or tweak data until it falls below it. This is usually not with bad intent at all, removing outliers is common for example. However, it’s also very human to want to see one’s hypothesis conformed after putting in a lot of time and effort to prove it [13].
Finally HARking, which means: Hypothesizing After the Results are Known. Especially in this day and age of lots of data, it’s easy to run the scientific process backwards. That is: start with some data and try to find significant differences based on slicing and dicing it in various ways. You may stumble upon a statistically significant result and based on that form an hypothesis. However, that result may just be noise. Since papers usually present both hypothesis and results, it’s hard to uncover whether this has happened in some form. This can also happen subtly, by adding an hypothesis or tweaking it after the results are already in.
The four systemic biases are much harder to reveal. Scientists may not be aware they are even practicing them. All of this leads to science exploring paths destined to fail. In short: lots of wasted effort, unreliable outcomes and damaged public perception.
Conclusion
I believe science is really the best way we have to come to objective conclusions. We can explain why things happen in a particular way, suggest how a specific challenge can be overcome and how to further improve an existing approach. However, that only works when everyone is in it for improving our objective understanding of the world around us and can actually overcome their own bias. We may not have set up the right incentive system to promote this.
Due to the sheer volume of academic research we have shifted heavily from assessing research on its inherent value, to assessing by proxies like reputation. This shifts attention of investors, researchers and the public to these proxies. This in turn provides an incentive to focus on just ‘increasing’ the proxy instead of focusing on research. We have seen this can lead to less creativity, novelty, and a focus on positive results: not what research should be about. We have also seen this triggers a spectrum of behaviors from subtle tweaking to outright fraud.
These unintended consequences take away from what scientific inquiry should be about: truth-finding driven by curiosity, fueled by creativity, leading to a world better understood. How can we change our system so that it provides the right incentives? What should we keep, what should we change? How can we create a world where scientist write when they have something to say, not when they have to say something?
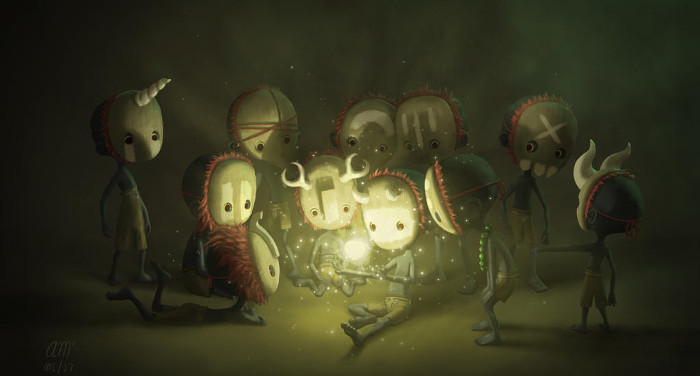
Sources
- Pink. D. H. (2009). Drive.
- Bishop, D. (2019). Rein in the four horsemen of irreproducibility.
- Wicherts, J. (2011). Psychology must learn a lesson from fraud case.
- Kreiner, G. (2016). The Slavery of the h-index – Measuring the Unmeasurable.
- An array of errors (2011). The Economist.
- Liar! Liar! (2009). The Economist.
- Olsthoorn, P. (2020). Diederik Stapel.
- Bhattacharjee, Y. (2013). The Mind of a Con Man.
- Retraction Watch (2021). Retraction Watch Leaderboard.
- Castelvechhi, D. (2021). Evidence of elusive Majorana particle dies.
- Brin, S. & Page. L (1998). The Anatomy of a Large-Scale Hypertextual Web Search Engine.
- Newcombe, R. G. (1987). Towards a reduction in publication bias.
- Aschwanden, C. (2019). We’re all ‘P-Hacking’ Now.